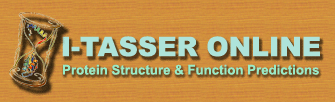
Introduction:
I-TASSER server is an Internet service for protein structure and function predictions.
Models are built based on multiple-threading alignments by LOMETS and iterative
TASSER simulations. I-TASSER (as 'Zhang-Server') was ranked as the No 1 server
in recent CASP7 and CASP8 experiments. The server is in active development with
the goal to provide accurate structural and function predictions using
state-of-the-art algorithms.
References:
- Ambrish Roy, Alper Kucukural, Yang Zhang.
I-TASSER: a unified platform for automated protein structure and function prediction.
Nature Protocols, vol 5, 725-738 (2010).
(download the PDF file).
- Yang Zhang. I-TASSER server for protein 3D structure prediction.
BMC Bioinformatics, vol 9, 40 (2008).
(download the PDF file).
|
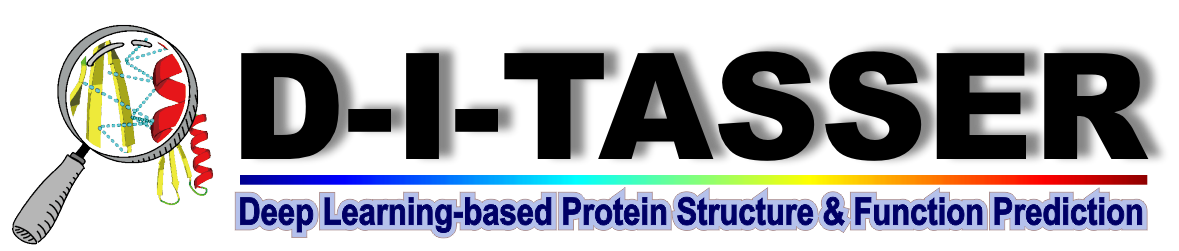
Introduction:
D-I-TASSER (Deep learning-based Iterative Threading ASSEmbly Refinement) is an advanced extension of I-TASSER
for high-accuracy protein structure and function prediction. It builds multiple sequence alignments (MSAs) via DeepMSA2,
predicts inter-residue distance/contact maps and hydrogen-bond networks using DeepPotential, AttentionPotential,
and optionally AlphaFold2, and identifies templates with LOMETS3.
Final models are assembled through Monte Carlo simulations guided by deep-learning restraints
and an improved domain-splitting module for modeling large multi-domain proteins.
D-I-TASSER ranked No. 1 in both single- and multi-domain categories in CASP15,
and outperforms AlphaFold2 and AlphaFold3 in accuracy benchmarks.
The server is free for all users, including commercial use,
with developer support available through its Discussion Board.
References:
- Wei Zheng, Qiqige Wuyun, Yang Li, Quancheng Liu, Xiaogen Zhou, Chunxiang Peng, Yiheng Zhu, Lydia Freddolino, Yang Zhang Deep learning-based single- and multi-domain protein structure prediction with D-I-TASSER. Nature Biotechnology, in press (2025).
|
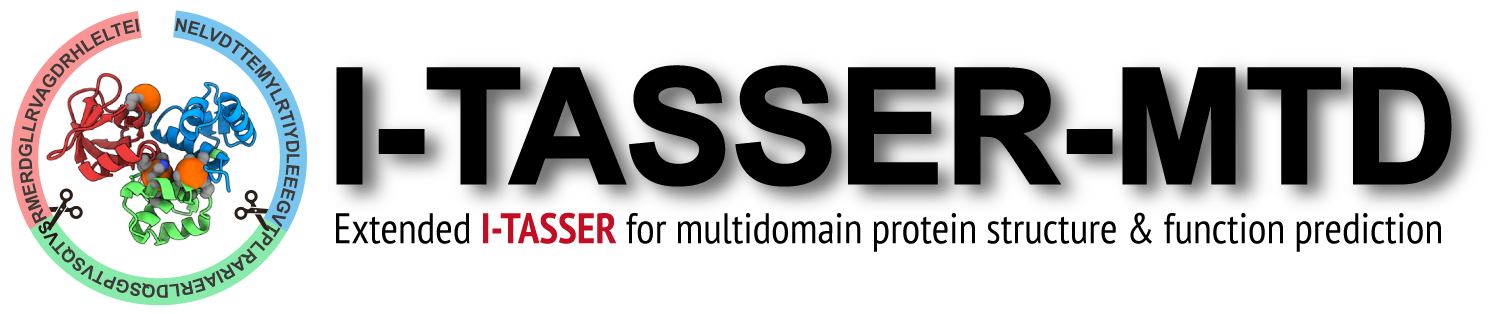
Introduction:
I-TASSER-MTD is multi-domain version of I-TASSER.
For a given sequence, it first predicts the domain boundaries by FUpred and ThreaDom
based on the deep-learning contact-map prediction and multiple threading alignments.
Next, the structure model of each individual domain is constructed independently by
I-TASSER guided by the deep learning predicted spatial restraints. Finally,
the individual domain models are assembled into full-length structure by DEMO
under guidance of quaternary structural templates and deep-learning distance profiles.
Meanwhile, the protein functions at both domain level and full-chain level are
annotated by COFACTOR based on structures, sequences, and protein-protein interaction networks.
References:
-
Xiaogen Zhou, Wei Zheng, Yang Li, Robin Pearce, Chengxin Zhang, Eric W. Bell, Guijun Zhang, and Yang Zhang. I-TASSER-MTD: A deep-learning based platform for multi-domain protein structure and function prediction, Nature Protocols, in press, 2022.
|
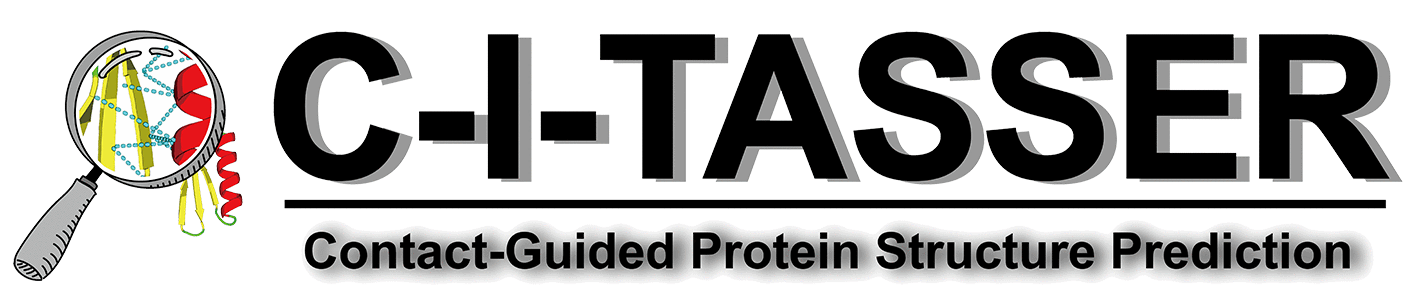
Introduction:
C-I-TASSER server is an extension of I-TASSER for contact-assisted
protein structure and function predictions.
By integrating deep-learning contact-maps, C-I-TASSER provides more
accurate structure predictions than I-TASSER,
especially for the targets that lack homologous templates in the PDB.
References:
-
Wei Zheng, Chengxin Zhang, Yang Li, Robin Pearce, Eric W. Bell, Yang Zhang
Folding non-homology proteins by coupling deep-learning contact maps with I-TASSER assembly simulations.
Cell Reports Methods, 1: 100014 (2021).
[PDF] [Support Information]
|
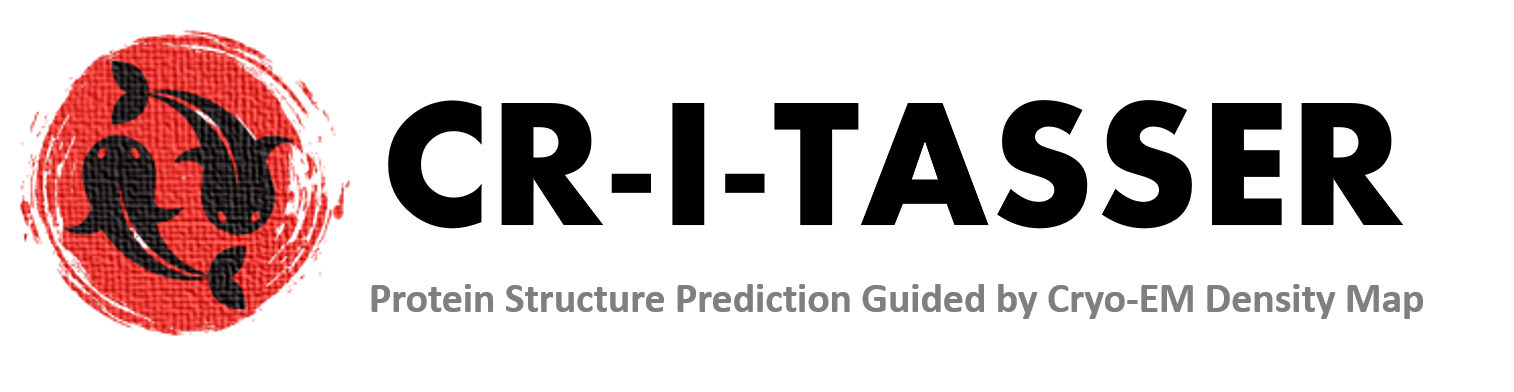
Introduction:
CR-I-TASSER is a hybrid method that integrates I-TASSER with cryo-EM density maps
for high-accuracy protein structure determination.
Starting from the density map, deep convolutional neural networks (CNNs)
predict C-alpha positions, which help improve template selection
via sequence-independent alignment and superposition.
The refined templates are then reassembled through I-TASSER-based simulations
to build full-length atomic models, guided by both density maps and template restraints.
Benchmark tests show that CR-I-TASSER significantly outperforms
existing de novo and refinement-based methods in cryo-EM modeling.
References:
- Xi Zhang, Biao Zhang, Peter L Freddolino, Yang Zhang. CR-I-TASSER: Assemble Protein Structures from Cryo-EM Density Maps using Deep Convolutional Neural Networks. Nature Methods, 19:195-204, 2022.
[PDF] [Support Information]
|

Introduction:
QUARK is a computer algorithm for ab initio protein folding and protein structure
prediction, which aims to construct the correct protein 3D model from amino acid
sequence only. QUARK models are built from a small fragments (1-20 residues long)
by replica-exchange Monte Carlo simulation under the guide of an atomic-level
knowledge-based force field. QUARK was ranked as the No 1 server in Free-modeling
(FM) in CASP9. Since no global template information is used in QUARK simulation,
the server is suitable for proteins which are considered without homologous templates.
References:
- D. Xu, Y. Zhang, Ab initio protein structure assembly using continuous
structure fragments and optimized knowledge-based force field. Proteins, 2012, 80: 1715-1735
(download the PDF file
and Support Information).
|
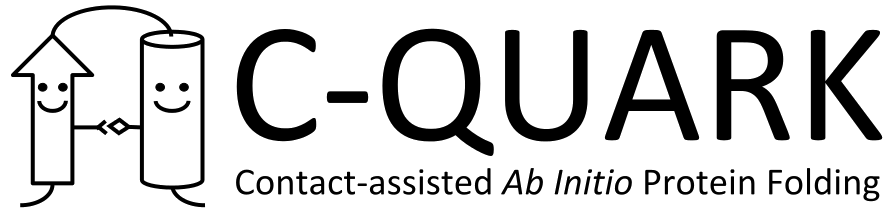
Introduction:
C-QUARK is an extension of QUARK for contact-assisted ab initio
protein folding and protein structure prediction.
By integrating deep-learning contact-maps, C-QUARK can provide more
accurate 3D structure modeling than QUARK for nearly all test cases.
References:
- S. M. Mortuza, Wei Zheng, Chengxin Zhang, Yang Li, Robin Pearce, Yang Zhang.
C-QUARK: Template-free protein structure modeling using low-accuracy contact-map
prediction. Nature Communications, in press, 2021.
|
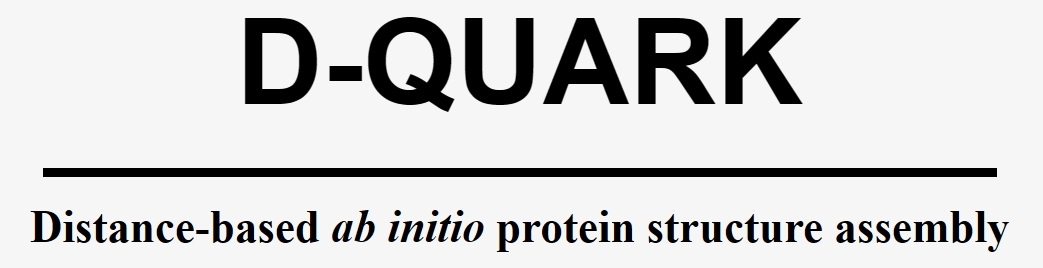
Introduction:
D-QUARK ('Distance-assisted QUARK') is an ab initio protein structure prediction method
that extends QUARK by incorporating deep-learning-based distance and orientation maps
into its fragment assembly simulations.
Starting from a query sequence, it builds a multiple sequence alignment (MSA) via DeepMSA2,
then predicts inter-residue distances and dihedral orientations using DeepPotential,
a deep residual convolutional network.
Local fragment structures are constructed through L-BFGS optimization,
and full-length models are assembled using replica-exchange Monte Carlo simulations,
guided by the predicted distance and orientation restraints.
D-QUARK ranked as the top automated server for FM targets in CASP14 under the "QUARK" group.
References:
- Chengxin Zhang, Yang Li, Yang Zhang (2021) D-QUARK: ab initio protein structure prediction guided by multiple deep learning predicted distance and orientation restraints.
|
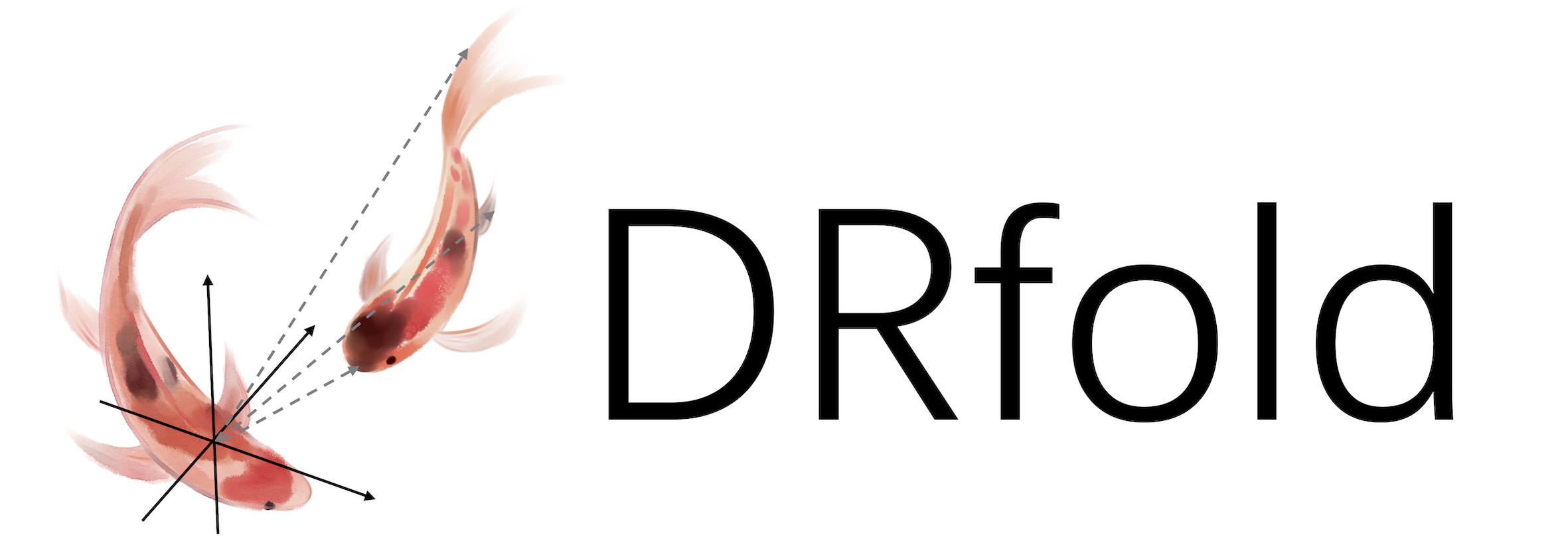
Introduction:
DRfold is a deep learning-based method for RNA tertiary structure prediction,
guided by end-to-end and geometry-based potentials.
Given a query sequence, it first extracts secondary structure features
as input to transformer networks, which predict rotation matrices and translation vectors for each nucleotide.
The predicted conformations are further optimized under a hybrid potential
that combines end-to-end and inter-residue geometry constraints.
References:
- Yang Li, Chengxin Zhang, Chenjie Feng, Robin Pearce, P. Lydia Freddolino, Yang Zhang. "Integrating end-to-end learning with deep geometrical potentials for ab initio RNA structure prediction." Nature Communications 14, no. 1 (2023): 5745.
[PDF] [Support Information]
|
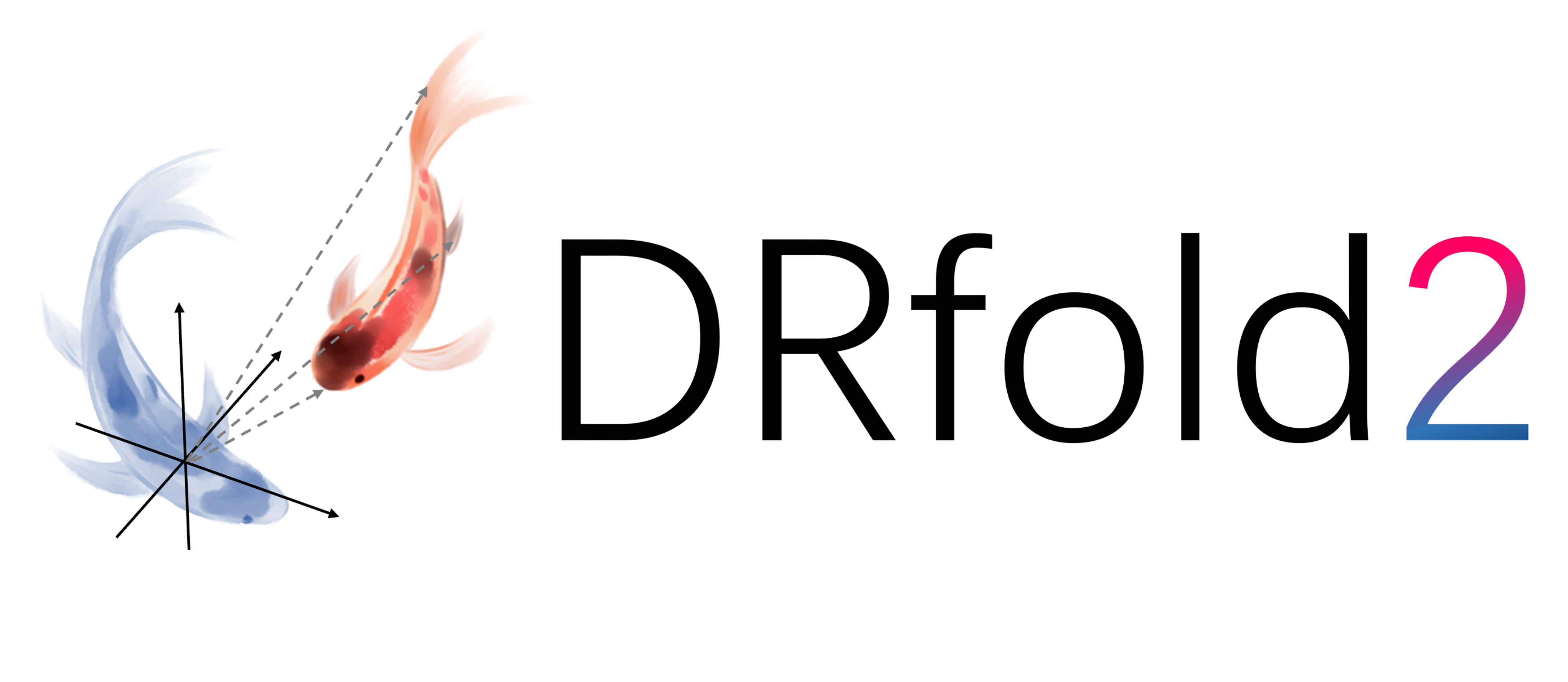
Introduction:
DRfold2 is an advanced RNA tertiary structure prediction method
that combines deep learning with a novel composite language model.
Given a query RNA sequence, it uses a pre-trained RNA Composite Language Model
to capture co-evolutionary patterns and secondary structure features.
Rotation matrices and translation vectors are predicted via end-to-end deep learning,
enabling accurate modeling of global topology and base pairing.
The structures are further refined through geometry-based optimization,
achieving up to 100% higher unsupervised contact precision than DRfold.
DRfold2 also complements AlphaFold3, offering statistically significant improvements
when combined via a hybrid optimization framework.
References:
- Yang Li, Chenjie Feng, Xi Zhang, Yang Zhang. "Ab initio RNA structure prediction with composite language model and denoised end-to-end learning", submitted.
|

Introduction: LOMETS (Local Meta-Threading-Server) is a locally installed
meta-server for protein structure prediction. It generates 3D models by collecting
consensus target-to-template alignments from 9 locally-installed threading programs
(FUGUE, HHsearch, PAINT, PPA-I, PPA-II, PROSPECT2, SAM-T02, SPARKS, SP3).
References:
- S. Wu, Y. Zhang.
LOMETS: A local meta-threading-server for protein structure prediction.
Nucleic Acids Research 2007; 35: 3375-3382
(download the PDF file).
|
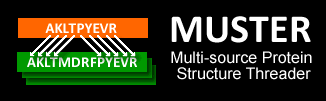
Introduction: MUSTER (MUlti-Sources ThreadER) is a new protein threading
algorithm to identify the template structures from the PDB library. It generate
sequence-template alignments by combining sequence profile-profile alignment
with multiple structural information.
References:
- S. Wu, Y. Zhang.
MUSTER: Improving protein sequence profile-profile alignments by using multiple sources of structure information.
Proteins: Structure, Function, and Bioinformatics 2008; 72: 547-556.
(download the PDF file)
|

Introduction:
CEthreader (Contact Eigenvector-based threader) is a threading method for protein
fold recognition. It enhances sequence-template alignment accuracy by integrating
sequence profile information with contact-map that is predicted from deep-learning.
References:
-
W Zheng, Q Wuyun, Y Li, SM Mortuza, C Zhang, R Pearce, J Ruan, Y Zhang.
Detecting distant-homology protein structures by aligning deep neural-network based contact maps.
PLOS Computational Biology, 15: e1007411 (2019).
[PDF]
[Support Information]
|

Introduction:
SEGMER is a segmental threading algorithm designed to recoginzing substructure motifs
from the Protein Data Bank (PDB) library. It first splits target sequences into segments
which consists of 2-4 consecutive or non-consecutive secondary structure elements
(alpha-helix, beta-strand). The sequence segments are then threaded through the PDB to
identify conserved substructures. It often identifies better conserved structure motifs
than the whole-chain threading methods, especially when there is no similar global fold
existing in the PDB.
References:
- S. Wu, Y. Zhang.
SEGMER:identifying protein sub-structural similarity by segmental threading. Structure, vol 18, 858-867 (2010).
(download the PDF file)
|
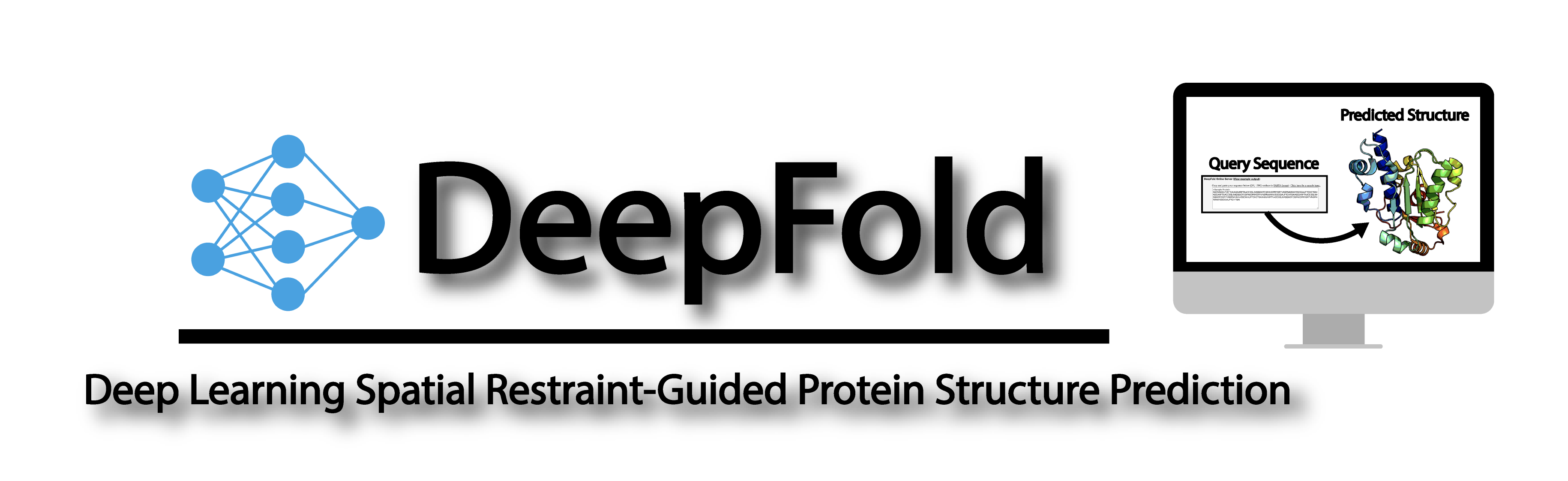
Introduction:
DeepFold is a deep learning-based ab initio protein structure prediction method.
Given a query sequence, it first builds multiple sequence alignments (MSAs)
from whole-genome and metagenome databases.
Spatial restraints—including contact maps, distance maps, and inter-residue orientations—are predicted
using DeepPotential, a convolutional residual neural network.
Final full-length structures are constructed through an L-BFGS folding algorithm.
References:
- Robin Pearce, Yang Li, Gilbert S. Omenn, Yang Zhang. Fast and Accurate Ab Initio Protein Structure Prediction Using Deep Learning Potentials, PLOS Computational Biology, 18: e1010539 (2022).
[PDF] [Support Information]
|
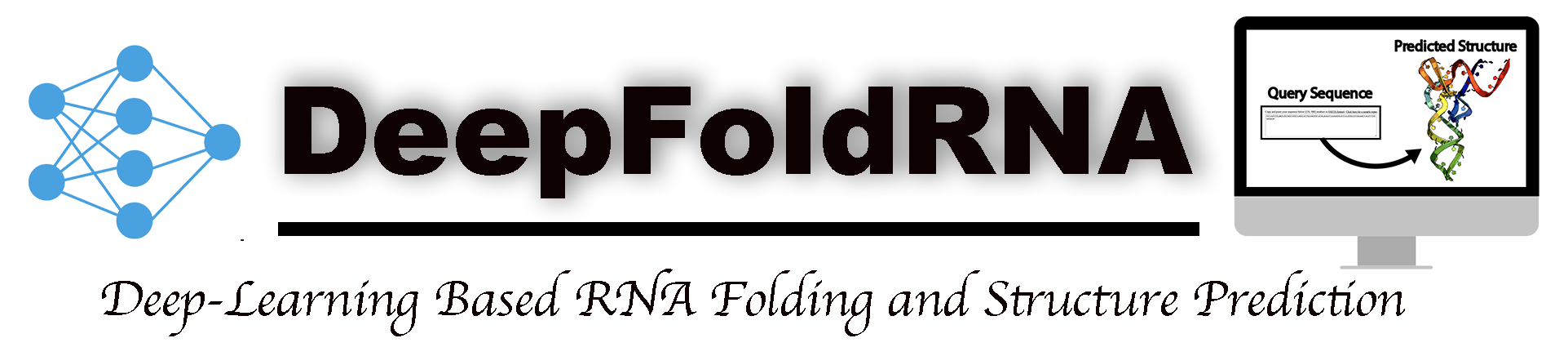
Introduction:
DeepFoldRNA is a deep learning-based method for de novo RNA tertiary structure prediction.
Given an RNA sequence, it first constructs homologous sequence alignments
from multiple sequence databases.
Spatial restraints—such as distance maps and inter-residue orientations—are predicted
using deep self-attention networks and transformed into negative log-likelihood potentials.
Final full-length structures are generated via L-BFGS simulations
by minimizing the potential with respect to backbone pseudo-torsion angles.
References:
- Robin Pearce, Gilbert S. Omenn, Yang Zhang. De novo RNA tertiary structure prediction at atomic resolution using geometric potentials from deep learning. BioRxiv, doi: https://doi.org/10.1101/2022.05.15.491755 (2022).
[PDF] [Support Information]
|
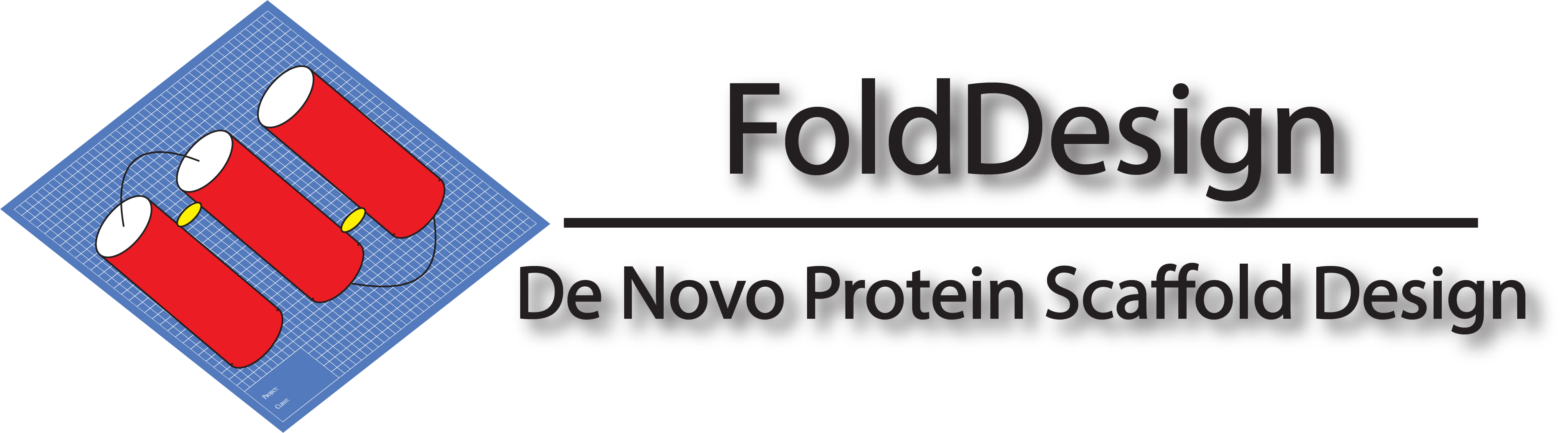
Introduction:
FoldDesign is a fragment-assembly-based method for de novo protein scaffold design.
Given user-defined constraints—such as secondary structure or contact/distance maps—
it first selects 1–20 residue fragments from the PDB that match the specified features.
Coarse-grained structural decoys are then generated via replica-exchange Monte Carlo simulations,
guided by a sequence-independent force field to satisfy the constraints.
The lowest-energy model from the largest structural cluster is selected,
followed by iterative atomic-level refinement and sequence design.
The final output includes both the designed sequence and its corresponding structural scaffold.
References:
- Pearce R, Huang X, Omenn G S, and Yang Zhang. De novo protein fold design through sequence-independent fragment assembly simulations[J]. Proceedings of the National Academy of Sciences, 120: e2208275120 (2022).
[PDF] [Support Information]
|
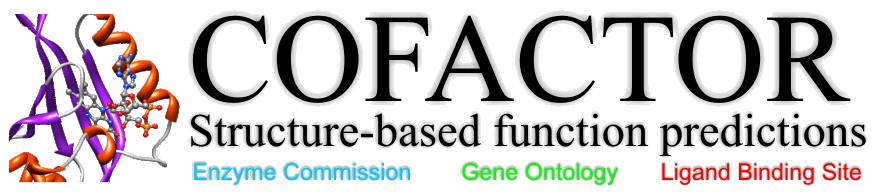
Introduction:
COFACTOR is an automated method for biological function annotation of protein
molecules, based on protein 3D structures. When user provides a structure model
of the target protein, COFACTOR will match the target proteins to the known
proteins (templates) in three comprehensive protein function libraries by global
and local structure comparisons. Functional insights, including ligand-binding site,
gene-ontology term, and enzyme classification, are then derived from the best
template proteins of the highest confidence score (C-score). The COFACTOR
algorithm was ranked as the best method for ligand-binding site predictions
in the community-wide CASP9 experiments.
References:
- Ambrish Roy, Jianyi Yang, and Yang Zhang.
COFACTOR: An accurate comparative algorithm for structure-based protein function annotation.
Nucleic Acids Research, 40:W471-W477 (2012). (download the PDF file)
- Ambrish Roy, Yang Zhang.
Recognizing protein-ligand binding sites by global
structural alignment and local geometry refinement.
Structure, 20: 987-997 (2012)
(download the PDF file and
Support Information)
- Chengxin Zhang, Peter L. Freddolino, Yang Zhang
COFACTOR: improved protein function prediction by combining structure,
sequence, and protein-protein interaction information.
Nucleic Acids Research, 45: W291-299 (2017).
(download the PDF file and
Support Information)
|

Introduction:
COACH is a meta-server approach to protein-ligand binding site prediction.
Starting from given structure of target proteins, COACH will generate
complementray ligand binding site predictions using two comparative methods,
TM-SITE and S-SITE, which recognize ligand-binding templates from the BioLiP
database by substructure and binding-specific sequence-profile comparisons.
These predictions will be combined with results from other methods
(including COFACTOR, FINDSITE and ConCavity to generate final ligand binding
site predictions. Users are also allowed to input primary sequence,
where I-TASSER will be used to generate 3D models first which are then
fed into the COACH pipeline for ligand-binding site prediction.
References:
-
Jianyi Yang, Ambrish Roy, and Yang Zhang. Protein-ligand binding site recognition using complementary binding-specific substructure comparison and sequence profile alignment,
Bioinformatics, 29:2588-2595 (2013).
[PDF]
[Support Information]
[Server]
|
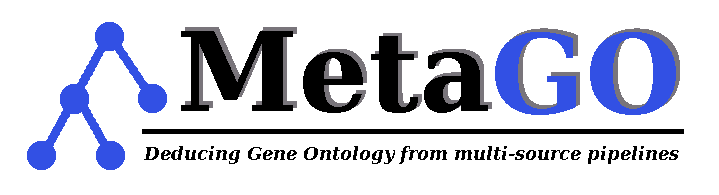
Introduction:
MetaGO is an algorithm for predicting Gene Ontology (GO) of proteins.
It consists of three pipelines to detect functional homologs through
local and global structure alignments, sequence and sequence profile
comparison, and parter's-homology based protein-protein interaction
mapping. The final function insights are a combination of the three
pipelines through logistic regression.
References:
- Chengxin Zhang, Peter L. Freddolino, and Yang Zhang.
MetaGO: Predicting Gene Ontology of non-homologous proteins through low-resolution protein structure prediction and protein-protein network mapping.
Journal of Molecular Biology, 430: 2256-2265 (2018).
[PDF]
[Support Information]
[Server]
|
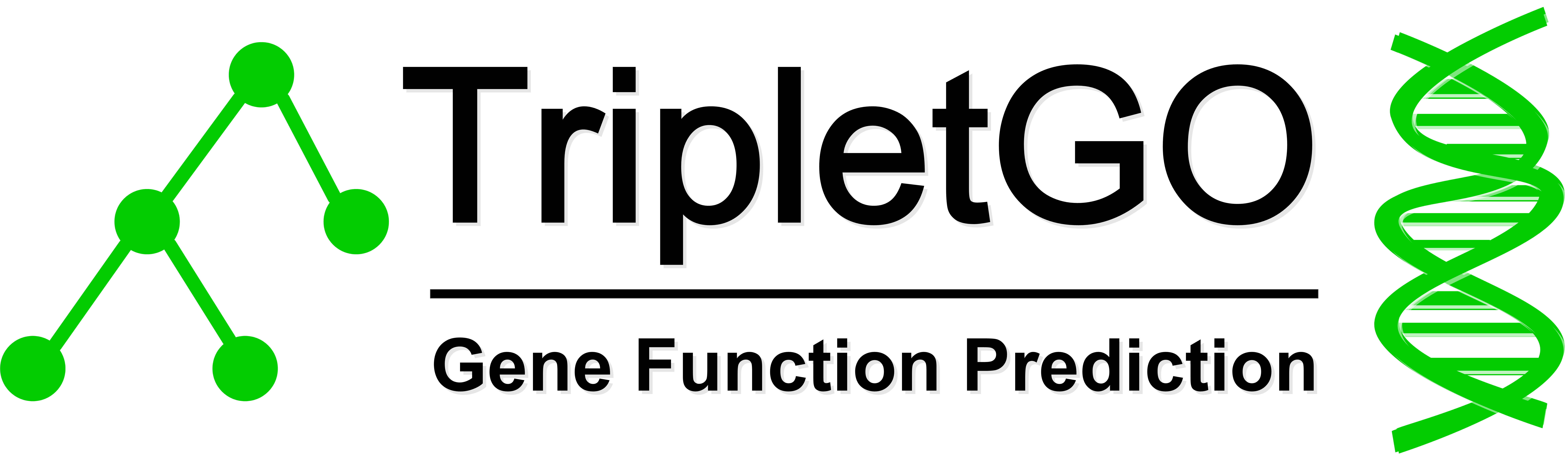
Introduction:
TripletGO is an algorithm for predicting Gene Ontology (GO) terms of genes
using a four-pipeline strategy.
It integrates (1) expression profile similarity via a triplet network,
(2) genetic sequence alignment, (3) protein sequence alignment,
and (4) naïve probability estimation.
Final GO predictions are obtained by combining outputs from all pipelines
using a neural network framework.
References:
- Yi-Heng Zhu, Chengxin Zhang, Yan Liu, Gilbert S. Omenn, Peter L. Freddolino, Dong-Jun Yu, Yang Zhang. Integrating transcript expression profiles with protein homology inferences for gene function prediction. Genomics, Proteomics & Bioinformatics, 20(5): 1013-1027 (2022).
[PDF] [Support Information]
|
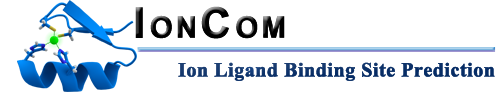
Introduction:
IonCom is an ligand-specific method for small ligand (including metal and acid radical ions) binding
site prediction. Starting from given sequences or structures of the query proteins, IonCom performs
a composite binding-site prediction that combines ab initio training and template-based transferals.
To enhance specificity and sensitivity, the server focuses on binding site prediction of thirteen most
important small ligand molecules, including nine metal ions (Zn++, Cu+, Fe+, Fe++, Ca++, Mg++, Mn++, Na+, K+)
and four acid radical ions (CO3--, NO2-, SO4--, PO4---).
References:
-
Xiuzhen Hu, Qiwen Dong, Jianyi Yang, Yang Zhang.
Recognizing metal and acid radical ion binding sites by integrating ab initio modeling with template-based transferals.
Boinformatics, 32: 3260-3269 (2016).
[PDF]
[Support Information] [Server]
|

Introduction:
FG-MD is a molecular dynamics (MD) based algorithm for high-resolution protein structure
refinement. Given an initial protein or protein complex 3D model (either in C-alpha or
full-atom), FG-MD first identifies analogous fragments from the PDB by the structural
alignment program TM-align. Spatial restraints extracted from the fragments are then
used to guide the molecular dynamics simulations. In general, FG-MD aims to refine
the initial models closer to the native structure. It also improves the local geometry
of the structures by removing the steric clashes and improving the torsion angle and
the hydrogen-binding networks.
References:
- Jian Zhang, Yu Liang, Yang Zhang. Atomic-Level Protein Structure Refinement
Using Fragment-Guided Molecular Dynamics Conformation Sampling. Structure, 19:
1784-1795, 2011 (Download the PDF file and the Support Information).
|

Introduction:
ModRefiner is an algorithm for atomic-level, high-resolution protein structure refinement. It can
start from either C-alpha trace, main-chain model or full-atomic model. Both side-chain and
backbone atoms are completely flexible during structure refinement simulations, where
conformational search is guided by a composite of physics- and knowledge-based force field.
ModRefiner has an option to allow for the assignment of a second structure
which will be used as a reference to which the refinement simulations are driven.
One aim of ModRefiner is to draw the initial starting models closer to their native state.
It also generates significant improvement in physical quality of local structures.
References:
- Dong Xu and Yang Zhang.
Improving Physical Realism and Structural Accuracy of Protein Models by
a Two-step Atomic-level Energy Minimization, Biophysical Journal, vol 101, 2525-2534 (2011)
(Download the PDF file).
|

Introduction:
REMO is a new algorithm for constructing protein atomic structures
from C-alpha traces by optimizing the backbone hydrogen-bonding networks.
References:
- Yunqi Li and Yang Zhang.
REMO: A new protocol to refine full atomic protein models from C-alpha traces by optimizing hydrogen-bonding networks.
Proteins, 2009, 76: 665-676.
(download the PDF file).
|
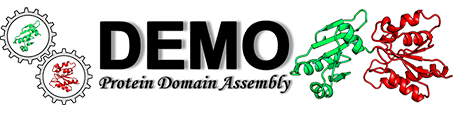
Introduction:
DEMO (Domain Enhanced MOdeling) is a method for automated assembly of full-length
structural models of multi-domain proteins, starting from individual domain structures.
References:
-
X Zhou, J Hu, C Zhang, G Zhang, Y Zhang.
Assembling multidomain protein structures through analogous global structural alignments.
Proceedings of the National Academy of Sciences, 116: 15930-15938 (2019). [PDF] [Support Information]
|

Introduction:
DEMO-EM is a hierarchical method for assembling multi-domain protein structures
from cryo-EM density maps.
Starting from a query sequence and cryo-EM data, individual domain structures
are predicted using FUpred, ThreaDom, and I-TASSER.
These domains are then assembled into full-length models through progressive rigid-body assembly
followed by atomic-level flexible refinement, guided by cryo-EM density and deep-learning distance profiles.
Benchmark tests demonstrate that DEMO-EM significantly outperforms
traditional homology-based methods in modeling multi-domain protein structures.
References:
- Xiaogen Zhou, Yang Li, Chengxin Zhang, Wei Zheng, Guijun Zhang, Yang Zhang. Progressive assembly of multi-domain protein structures from cryo-EM density maps. Nature Computational Science, 2: 265-275 (2022).
[PDF] [Support Information]
|
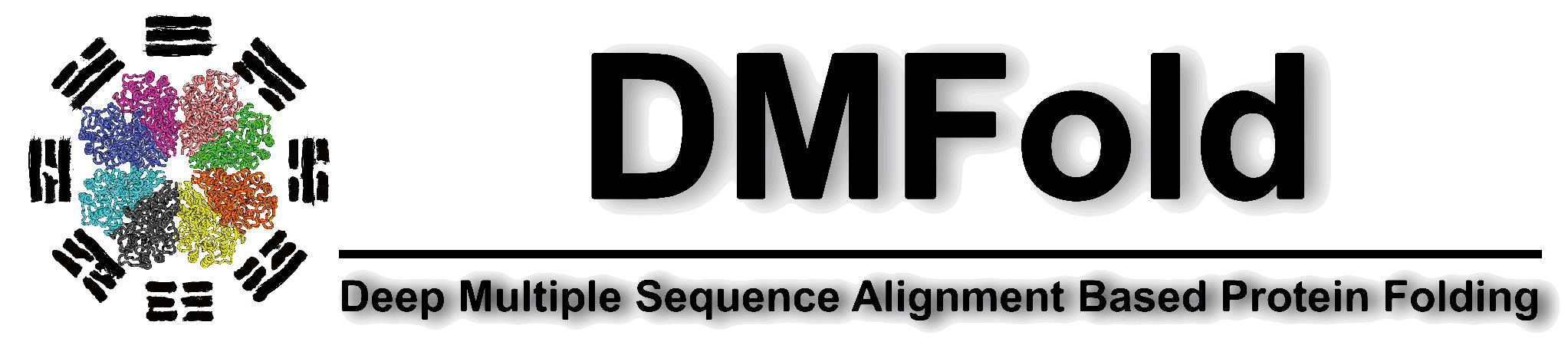
Introduction:
DMFold (also known as DMFold-Multimer) is a deep learning-based method
for protein complex structure and function prediction, built on deep multiple sequence alignments (MSAs).
It integrates DeepMSA2 with a modified AlphaFold2-Multimer module.
Starting from query sequences, it generates deep monomeric MSAs through iterative searches
across whole-genome (Uniclust30, UniRef90) and metagenome (Metaclust, BFD, Mgnify, TaraDB, MetaSourceDB, JGIclust) databases.
Multimeric MSAs are then constructed by pairing monomeric MSAs based on species annotations.
Complex structures are predicted using AlphaFold2-Multimer, and functional annotations
(Gene Ontology, Enzyme Commission, Ligand Binding Sites) are provided by COFACTOR2 and US-align.
DMFold ranked No. 1 for PPI complex prediction in CASP15,
outperforming AlphaFold2-Multimer (NBIS-AF2-multimer) in accuracy.
The server also supports single-chain modeling via the 'DMFold-Monomer' pipeline,
and is freely available to all users, including commercial use.
Please report issues on the Zhang Lab Server Forum for developer support.
References:
- Wei Zheng, Qiqige Wuyun, Yang Li, Chengxin Zhang, P Lydia Freddolino, Yang Zhang. Improving deep learning protein monomer and complex structure prediction using DeepMSA2 with huge metagenomics data. Nature Methods, 21: 279-289 (2024).
[PDF] [Support Information]
|

Introduction:
SPRING is a template-base algorithm for protein-protein structure prediction.
It first threads one chain of the protein complex through the PDB library
with the binding parters retrieved from the original oligomer entries.
The complex models associated with another chain is deduced from a pre-calculated
look-up table, with the best orientation selected by the SPRING-score which is
a combination of threading Z-score, interface contacts, and TM-align match between monomer-to-dimer templates.
References:
- Aysam Guerler, Brandon Govindarajoo and Yang Zhang. Mapping monomeric threading to protein-protein structure prediction, Journal of Chemical Information and Modeling 2013, 53: 717-725.
(Download the PDF file).
|
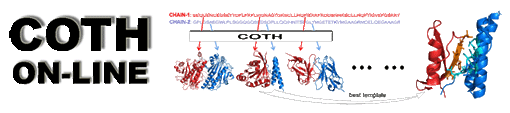
Introduction:
COTH (CO-THreader) is a multiple-chain protein threading algorithm to identify
and recombine the protein complex structures from both tertiary and complex
structure libraries. It first generates complex query-template alignments by
sequence profile-profile alignment assisted by the ab initio binding-site
predictions from BSpred. The monomer structures from tertiary template library
are then combined into the complex framework by structure superposition.
References:
- S Mukherjee, Y Zhang
Protein-protein complex structure prediction by multimeric threading and template recombination.
Structure, vol 19, 955-966 (2011)
(Download the PDF file
and Supporting Information).
|
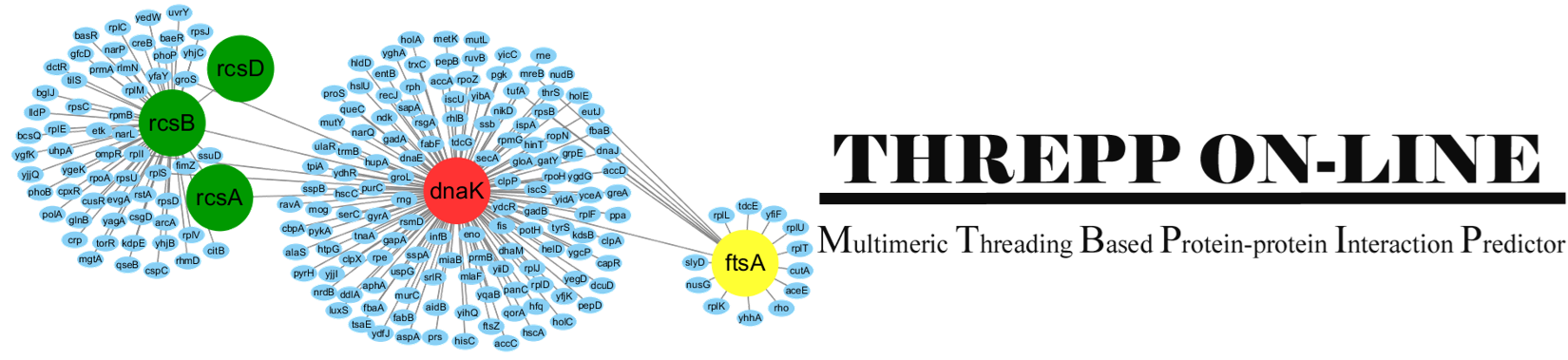
Introduction:
Threpp is a method for protein-protein interaction (PPI) prediction.
Starting from a pair of protein sequences, it does two things:
(1), it will judge whether the two proteins interact with each other by calculating
the likelihood through a naive Bayes classifier model which combines the Threpp threading score
and available high-throughput experimental (HTE) data.
(2), it creates the quaternary stuctural models of the PPIs by reassembling
the monomeric threading templates with the identified PPI frameworks.
References:
- Weikang Gong, Aysam Guerler, Chengxin Zhang, Elisa Warner, Chunhua Li, Yang Zhang.
Integrating Multimeric Threading With High-throughput Experiments for Structural Interactome of Escherichia coli
.
Journal of Molecular Biology, 433: 166944 (2021).
[PDF]
[Supporting Information]
|
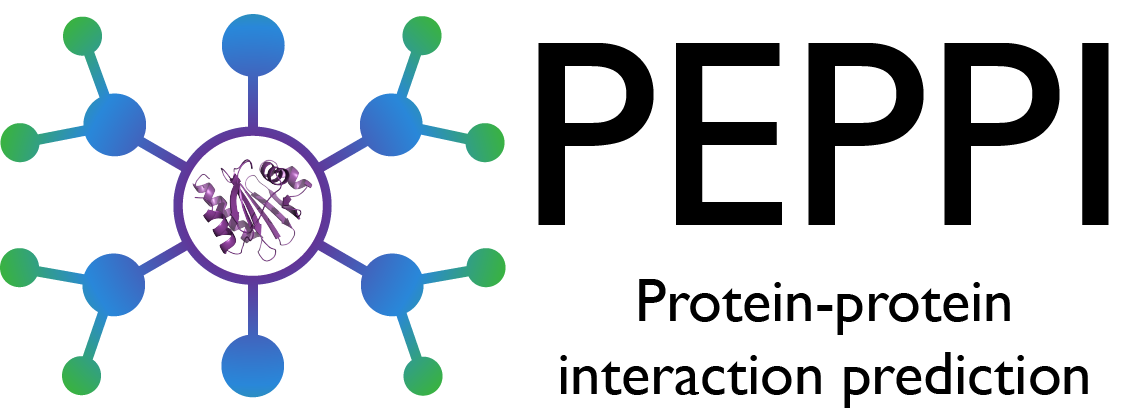
Introduction:
PEPPI (Pipeline for the Extraction of Predicted Protein-protein Interactions)
is a computational method for predicting direct physical interactions between proteins.
Given a pair of amino acid sequences, it evaluates interaction likelihood
using multiple independent approaches: multimeric threading for structural homology,
BLAST search against high-throughput datasets for sequence homology,
functional association via STRING, and machine learning-based classification.
These scores are combined through a naïve Bayesian model
to produce a final likelihood ratio reflecting the probability of interaction versus non-interaction.
References:
- E.W. Bell, J.H. Schwartz, P.L. Freddolino, Y. Zhang. PEPPI: Whole-proteome protein-protein interaction prediction through structure and sequence similarity, functional association, and machine learning. Journal of Molecular Biology, 167530 (2022).
[PDF] [Support Information]
|
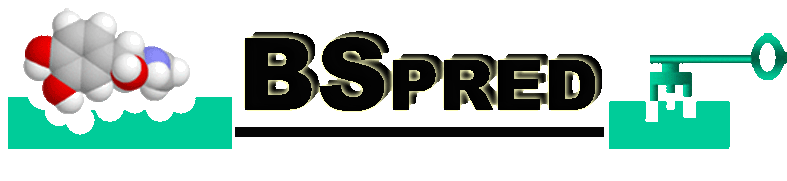
Introduction:
BSpred is a neural network based algorithm for predicting binding site of proteins
from amino acid sequences. The algorithm was extensively trained on the sequence-based
features including protein sequence profile, secondary structure prediction,
and hydrophobicity scales of amino acids.
References:
- S Mukherjee, Y Zhang
Protein-protein complex structure prediction by multimeric threading and template recombination.
Structure, vol 19, 955-966 (2011)
(Download the PDF file
and Supporting Information).
|
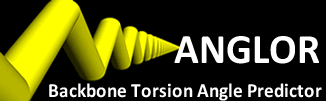
Introduction:
ANGLOR is a machine-learning based algorithm for ab initio prediction
of protein backbone torsion angles. For a given amino acid sequence,
the real-value backbone torsion angles (phi and psi) for each residue
are predicted by the combination of the neural network training and
the support vector machine.
References:
- S. Wu, Y. Zhang.
ANGLOR: A Composite Machine-Learning Algorithm for Protein Backbone Torsion Angle Prediction.
PLoS ONE 2008; 3: e3400.
(download the PDF file)
|
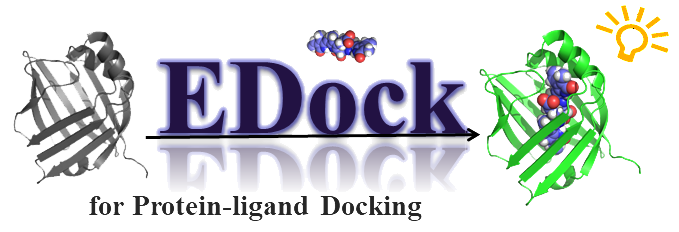
Introduction:
EDock is method for blind ligand-protein docking.
It starts with initial ligand poses generated by a modified graph matching on the predicted binding pockets.
Replica-exange Monte Carlo (REMC) simulations are then performed for ligand conformation sampling
under the guidance of a physical force field coupled with binding site constraints.
The final ligand docking model is selected by a composite knowledge-based score function.
References:
-
Wenyi Zhang, Eric Bell, Minghao Yin, Yang Zhang.
EDock: Blind Protein-ligand Docking by Replica-Exchange Monte Carlo Simulation.
Journal of Cheminformatics, 12: 37 (2020).
[PDF]
[Support Information]
[Server]
|

Introduction:
BSP-SLIM is a blind molecular docking method on low-resolution protein structures.
The method first identifies putative ligand binding sites by structurally matching the
target to the template holo-structures. The ligand-protein docking conformation is then
constructed by local shape and chemical feature complementarities between ligand
and the negative image of binding pockets.
References:
- Hui Sun Lee and Yang Zhang. BSP-SLIM: A blind low-resolution ligand-protein docking approach using theoretically predicted protein structures, Proteins, 2012, 80:93-110
(download the PDF file).
|
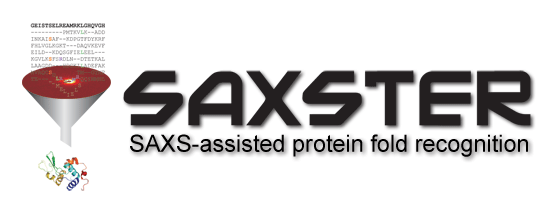
Introduction:
SAXSTER is a new algorithm to combine small-angle x-ray scattering (SAXS) data and
threading for high-resolution protein structure determination. Given a query sequence,
SAXSTER first generates a list of template alignments using the MUSTER threading program
from the PDB library. The SAXS data will then be used to prioritize the best template
alignments based on the SAXS profile match, which are finally used for full-length
atomic protein structure construction.
References:
- M. dos Reis, R. Aparicio and Y. Zhang.
Improving protein template recognition by using small angle X-ray scattering profiles.
Biophysical Journal, vol 101, 2770-2781 (2011)
(Download the PDF file).
|
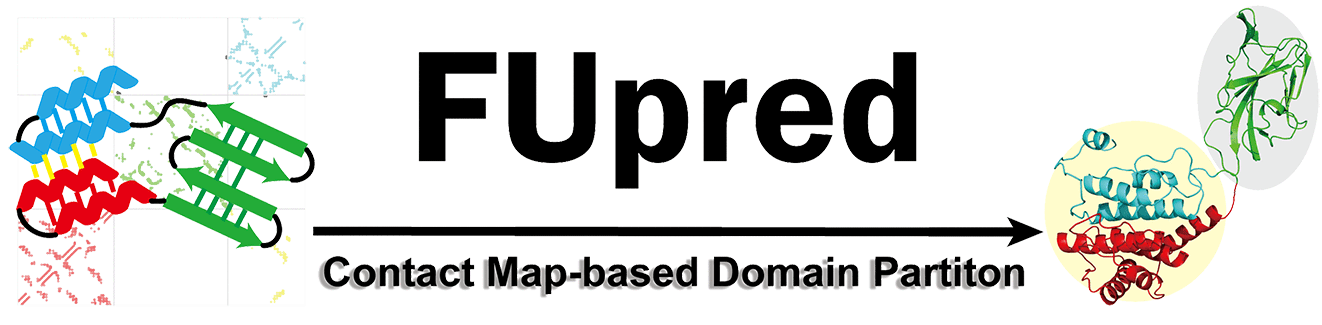
Introduction:
FUpred is a contact map-based protein domain prediction method.
It utilizes a recursion strategy to detect domain boundary based on predicted contact-map
and secondary structure information.
References:
-
Wei Zheng, Xiaogen Zhou, Qiqige Wuyun, Robin Pearce, Yang Li, Yang Zhang
FUpred: Detecting protein domains through deep-learning based contact map prediction.
Bioinformatics, 36: 3749–3757 (2020).
[PDF]
[Support Information]
[Server]
|

Introduction:
ThreaDom is a template-based algorithm for protein domain boundary prediction.
Given a protein sequence, ThreaDom first threads the target through the PDB library
to identify protein template that have similar structure fold.
The domain boundary is then assigned based on the multiple sequence alignment
between target and template structures, where a confidence score is assigned to
each prediction which combines information from template structure, terminal and
internal gaps and insertions. ThreaDom is designed to predict both continuous and discontinuous domains.
References:
- Z Xue, D Xu, Y Wang, Y Zhang. ThreaDom: Assigning protein domain boundary using multiple threading alignments.
Bioinformatics, 29: i247-i256, 2013.
[PDF]
[Server]
|

Introduction:
ThreaDomEx is a new version of template-based domain prediction program, which is
extended from ThreaDom. Compared to the ThreaDom program, the major new features
in ThreaDomEx include:
(1) it enables discontinuous domain prediction;
(2) it allows manual intervention of domain prediction.
References:
- Yan Wang, Jian Wang, Ruiming Li, Qiang Shi, Zhidong Xue, Yang Zhang.
ThreaDomEx: a unified platform for predicting continuous and discontinuous protein domains by multiple-threading and segment assembly.
Nucleic Acids Research, 45: W400-W407, (2017).
[PDF] [Server]
|

Introduction:
EvoDesign is an evolutionary profile based approach to de novo protein
design. Starting from a scaffold of target protein structure, EvoDesign
first identifies protein families which have similar fold from the PDB
library by TM-align. A structural profile is then constructed from the
protein templates which is used to guide the conformation search of amino
acid sequence space, where physicochemical packing is accommodated by the
single-sequence based solvation, torsion angle and secondary structure
predictions. The final designed sequence is obtained by clustering all
sequence decoys generated during design simulations.
References:
- Pralay Mitra, David Shultis and Yang Zhang. EvoDesign: de novo protein design based on structural and evolutionary profiles. Nucleic Acids Research, W273-W280, 2013.
[PDF]
[Support Information] [Server]
|
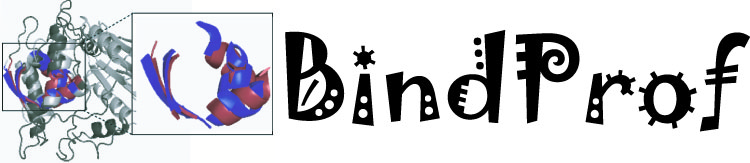
Introduction:
BindProf is a method for predicting free energy changes (ÎÎG) of protein-protein binding
interactions upon mutations of residues at the interface.
While BindProf adopts a multi-scale approach using multiple sources of information at
different levels of structural resolution, a unique feature of BindProf is the inclusion
of an interface structural profile score derived from multiple structure alignments
from analogous protein-protein interactions.
References:
-
Jeffrey R. Brender, Yang Zhang.
Predicting the Effect of Mutations on Protein-Protein Binding Interactions through Structure-Based Interface Profiles.
PLOS Computational Biology, 11: e1004494 (2015).
[PDF]
[Support Information].
|
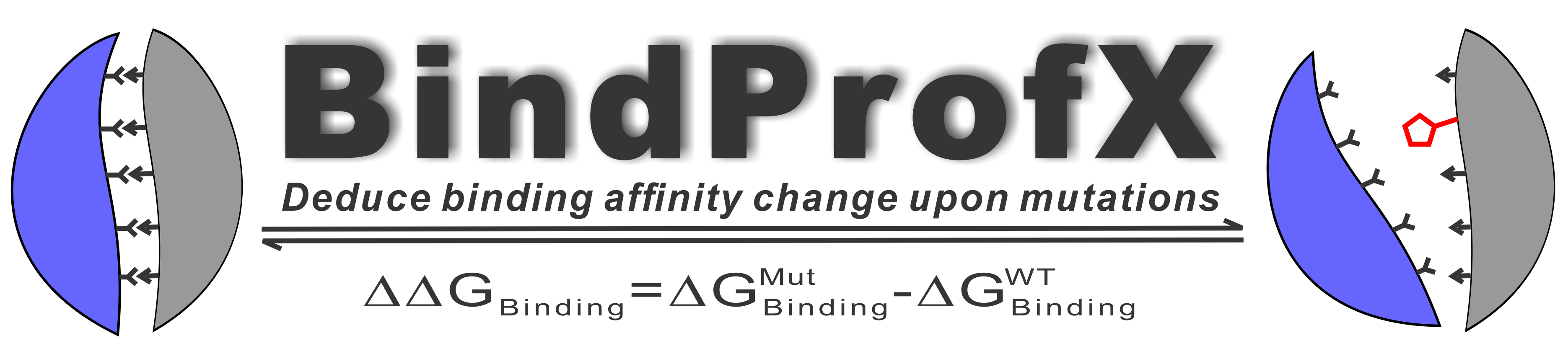
Introduction:
BindProfX is a method to assess protein-protein binding free-energy
changes (ÎÎG) induced by single- and multiple-mutations. This is an update on
the BindProf method and tries to enhance PPI ÎÎG prediction accuracy using
log-odds likelihood and pseudo count techniques.
References:
-
P Xiong, C Zhang, W Zheng, Y Zhang.
BindProfX: Assessing mutation-induced binding affinity change by protein interface profiles with pseudo counts.
J Mol Biol. 429: 426-434, 2017.
[PDF]
[Supplementary Information].
|
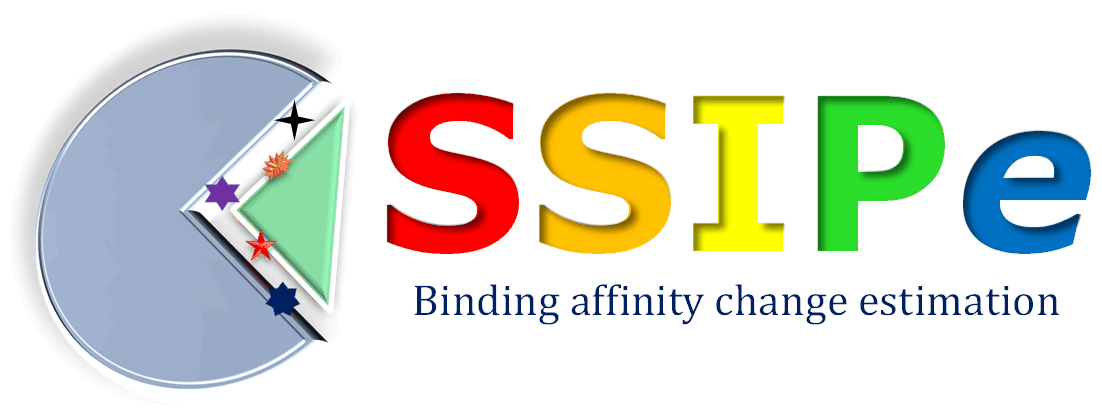
Introduction:
SSIPe is a method to calculate binding affinity changes (ÎÎG) of
protein-protein interactions (PPIs) upon mutations at protein-protein interface.
The method is a significant extension of BindProf/BindProfX by integrating
PPI interface structural profiles with sequence profiles and physics-based
physical energy function EvoEF.
References:
-
X Huang, W Zheng, R Pearce, Y Zhang.
SSIPe: accurately estimating protein-protein binding affinity change upon mutations
using evolutionary profiles in combination with an optimized physical energy function.
Bioinformatics, 36: 2429-2437 (2020). [PDF] [SI] [Server]
|
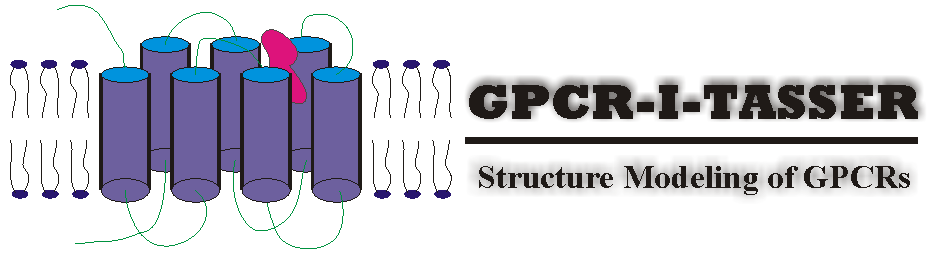
Introduction:
GPCR-I-TASSER is on-line server system specfically designed for
predicting 3D structure of G protein-coupled receptors.
The target sequence is first threaded through
the PDB libary by LOMETS to search for putative templates. If homologous
templates are identified, a template-based fragment assembly procedure is
used to construct full-length models.
In case that no homologous templates are available, an ab initio
TM-helix folding procedure is used to assembly the 7-TM-helix bundle
from scratch, followed by GPCR-I-TASSER structure reassembly simulation
assisted with the sparse mutagensis restraints from GPCR-RD. The final
structue models are refined at atomic-level by the fragment-guided molecular dynamic
(FG-MD) simulations.
References:
-
Jian Zhang, Jianyi Yang, Richard Jang, Yang Zhang.
GPCR-I-TASSER: A hybrid approach to G protein-coupled receptor structure modeling and the application to the human genome.
Structure, 23: 1538-1549 (2015).
[PDF] [Support Information]
[Server]
[Database]
|
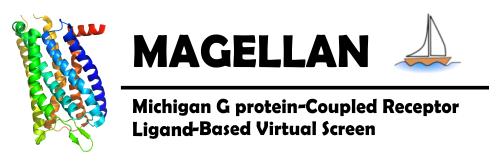
Introduction:
MAGELLAN (Michigan G protein-coupled Receptor Ligand-Based Virtual Screen)
is a ligand-based virtual screening pipeline developed for screening Class-A G protein-coupled receptors (GPCR).
The core of this pipeline is the construction of a composite ligand profile, represented by 1024xN matrix,
that is collected from homologous ligand-GPCR interactions detected by sequence and structure alignments.
Active GPCR compounds are then prioritized by threading the ligand profile through large-scale compound
databases.
References:
-
Wallace K.B. Chan, Yang Zhang.
Virtual screening of human Class-A GPCRs using ligand profiles built on multiple ligand-receptor interactions.
Journal of Molecular Biology, 432: 4872-4890 (2020).
[PDF]
[Support Information]
|
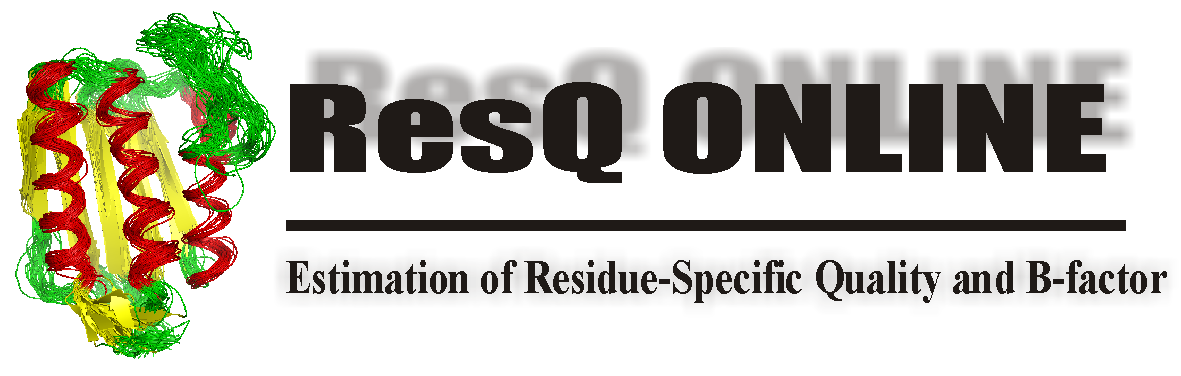
Introduction:
ResQ is a method for estimating B-factor and residue-level quality in protein
structure prediction, based on local variations of modelling simulations and
the uncertainty of homologous alignments. Given a protein structure model, ResQ
identifies a set of homologous and/or analogous templates from the PDB by
threading and structure alignment techniques. The residue-level modeling errors
are then derived by support vector regression, with the B-factor of each residue
deduced from the experimental records of the top homologous proteins.
References:
-
Jianyi Yang, Yan Wang, Yang Zhang.
ResQ: An approach to unified estimation of B-factor and residue-specific error in protein structure prediction.
Journal of Molecular Biology, 428: 693-701 (2016).
[PDF]
[Support Information] [Server]
|
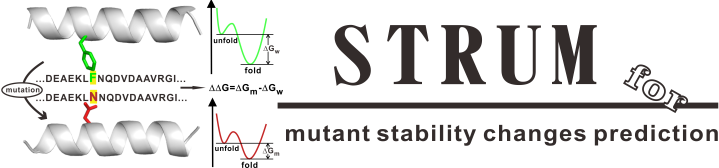
Introduction:
STRUM is a method for predicting the fold stability change (ÎÎG) of protein molecules upon single-point nsSNP
mutations. STRUM adopts a gradient boosting regression approch to train the Gibbs free-energy changes
on a variety of features at different levels of sequence and structure properties. The unique characteristics
of STRUM is the combination of sequence profiles with low-resolution structure models from protein structure
prediction, which helps enhance the robustness and accuracy of the method and make it applicable to various
protein seqences, including those without experimental structures.
References:
-
Lijun Quan, Qiang Lv, Yang Zhang.
STRUM: Structure-based stability change prediction upon single-point mutation,
Boinformatics, 32: 2936-46 (2016).
[PDF]
[Support Information]
[Server]
|
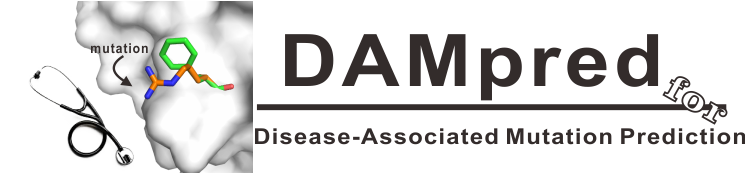
Introduction:
DAMpred is a method to predict what gene mutations can cause human diseases and what
mutations do not do so. Starting with a protein sequence and specified non-synonymous
single nucleotide polymorphisms (nsSNPs), DAMpred calculates the probability of the
mutations to be deleterious or neutral to human health. The calculation is built on
a deep-learning model that integrates three sources of information from sequence
profiles, biological assembly and 3D structure model (by I-TASSER), which is trained
through a novel Bayes-guided artificial neural network (BANN) algorithm.
References:
-
Lijun Quan, Hongjie Wu, Qiang Lyu, Yang Zhang.
DAMpred: Recognizing disease-associated nsSNPs through Bayes-guided neural-network
model built on low-resolution structure prediction of proteins and protein-protein
interactions.
J Mol Biol, 431: 2449-2459 (2019).
[PDF] [Support Information]
[Server]
|
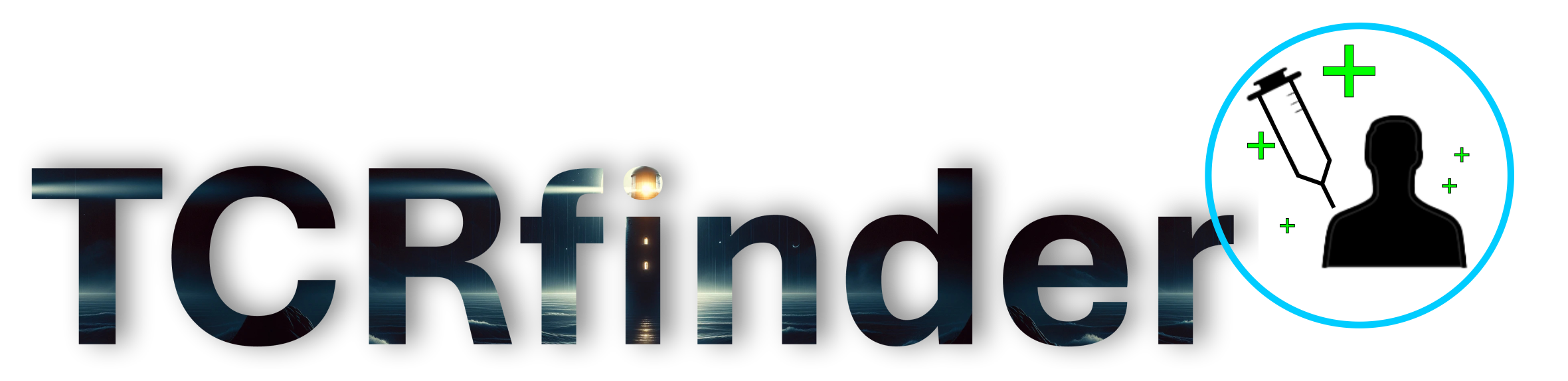
Introduction:
TCRfinder is a deep learning pipeline for sequence-based screening of T-cell receptors (TCRs) and neoantigens.
It focuses on predicting TCR-peptide interactions using the β-chain CDR3 region and peptide sequences.
The pipeline begins with pre-training two separate language models for TCRs and peptides,
which are then concatenated into a joint embedder model.
Transformer blocks are applied to both paired and individual representations,
followed by a Multi-Layer Perceptron (MLP) to score interaction likelihoods.
To address both prediction directions, TCRfinder trains two distinct models:
one for peptide-based TCR screening and another for TCR-based neoantigen screening.
References:
- Yang Li, Chaoting Zhang, Xi Zhang, Yang Zhang. TCRfinder: Improved TCR virtual screening for novel antigenic peptides with tailored language models, submitted (2024).
|
|
|